Research
Stoichiometric Determinants of Metabolic Network States
The stoichiometric description of metabolic networks lends itself to direct, physical interpretations of the four fundamental subspaces of the mass balance equations. The right and left null spaces contain information about steady state capabilities of the biochemical network. The row and column spaces account for the network dynamics. High- and low-throughput data can be used to further constrain these networks and improve the sensivity and specificity of network predictions.
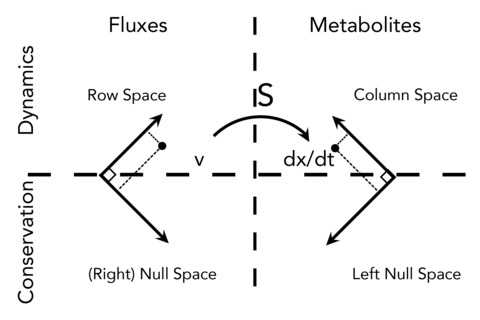
Drug Response Phenotypes: Assessment of altered platelet flux states in aspirin resistance
As an example of how genome-scale models can be used for precision medicine applications using multi-omics data. Although aspirin is a commonly taken pharmaceutical and frequently prescribed as a ‘first line’ prophylactic medication for clotting disorders, it is surprisingly estimated 10-20% of individuals may have diminished response to inhibition of cyclooxygenase (aspirin’s target).
We developed a platelet reconstruction, iAT-PLT- 636, to assess the cellular alterations in subjects who have normal versus abnormal (‘resistant’) responses to aspirin. Findings from this work suggested that the metabolic consequences of aspirin-resistance on platelet metabolism are elicited by the diversion of metabolites to prostaglandin synthesis and the consequent flux decrease in oxidative metabolism and flux increase in the pentose sugar metabolism with corresponding shifts in redox pathways, glycolysis, and nucleotide salvage pathways.
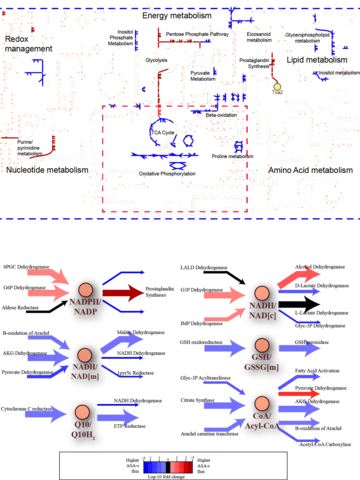
Radiogenomic Map for Pancreatic Cancer:
Radiogenomics is a top-down approach for non-invasive (or minimally invasive) genotyping different disease states by integrating clinical imaging data with cellular, omics data in order to help diagnose, classify, and guide treatment strategies for complex diseases.
We performed a radiogenomic (RG) analysis to construct CT-imaging feature to transcriptome association maps for pancreatic adenocarcinoma (PDAC).
Current work in the SIMLab is focused on building up the bottom-up characterization of these maps and to establish links (if they exist) between between bottom-up and top-down assessments of PDAC.
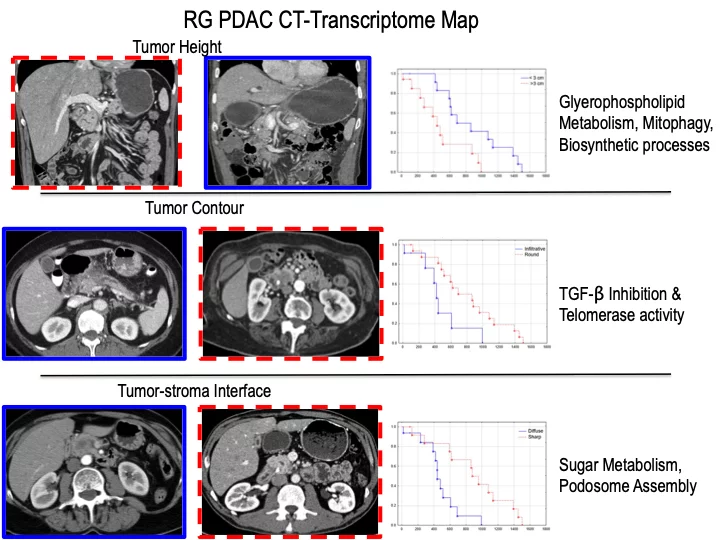
Multi-Modality Characterization of Pediatric Non-Alcoholic Fatty Liver Disease
Non-alcoholic fatty liver disease has become a significnat health problem with reports as high as 34% in pediatric obesity clinics. We constructed a multi-modality correlation network based upon histopathology, clinical blood profiles, cross-sectional imaging, and metabolomics in a pediatric cohort. These multi-modality maps could serve as a framework for characterizing disease status and progression and could potentially guide medical interventions. Future work is focused on drilling down to more mechanistic, cellular-metabolic phenotypic characterization of these network associations.
